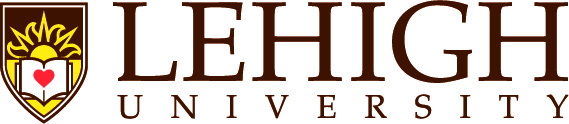
Image by iStock/adventtr
In this episode of Lehigh University’s College of Business ilLUminate podcast, we're talking with Rebecca Wang about a recent study she conducted with colleagues at Lehigh University and Seattle University that suggests highlighting human bias can reduce individuals' resistance to the use of artificial intelligence, or AI, in health care.
Wang holds the Class of '61 Professorship in marketing in Lehigh's College of Business. She was the lead author on the study titled “To err is human: Bias salience can help overcome resistance to medical AI,” which was published recently in the journal Computers and Human Behavior.
The study was co-authored by Matthew Isaac of the Albers School of Business and Economics at Seattle University; Lucy Napper, associate professor of psychology, director of undergraduate studies, and associate director of the Health, Medicine and Society Program in Lehigh's College of Arts and Sciences; and Jessecae Marsh, professor of psychology and associate dean for interdisciplinary programs and international initiatives in Lehigh's College of Arts and Sciences.
Wang’s research reflects her interest in marketing, data science, and technologies, and focuses on digital and mobile channels, social media dynamics, and data-driven marketing strategies.
She spoke with Jack Croft, host of the ilLUminate podcast. Listen to the podcast here and subscribe and download Lehigh Business on Apple Podcasts or wherever you get your podcasts.
Below is an edited excerpt from that conversation. Read the complete podcast transcript [PDF].
Jack Croft: Cutting to the chase, with the realization that the title of the study kind of is a spoiler alert for the overall finding, what were some of the other key findings from your study?
Rebecca Wang: The findings from this paper are quite straightforward. Half of it is in the title. So, essentially, we show that bias salience reduces patients' resistance to medical AI. In other words, reminding people that biases exist and are inherent in humans' decision-making process can make them more receptive to AI in health care settings.
This effect of bias salience on reducing resistance to AI was consistently found across multiple studies, across multiple variations in medical scenarios, as well as the types of biases highlighted. For instance, just general cognitive bias or gender bias or age bias. And we also show that bias salience increases perceived AI integrity. So high bias salience not only reduced patients' resistance toward AI, but it also enhances the perceived integrity of AI. So participants, in other words, view AI as more fair and more trustworthy when they're reminded that humans, in fact, are biased in nature.
Croft: You talked about multiple studies. And without getting too deep into the weeds about how each was conducted, I think it would be helpful to talk about the six studies you and your colleagues conducted and what they revealed about bias salience.
Wang: First, we started out with a preliminary pilot study that assessed whether people associate bias more with human providers than with AI. And the results confirmed what we suspected, which is robots don't judge, right? Patients-- or participants, to be specific, in fact, perceived human providers to be more biased than AI.
So this finding sets the stage for our later studies. Then we conducted four experimental studies to test whether our hypothesis, which is high bias salience—in other words, making participants become more aware of human biases—could, in fact, influence their preference for medical AI versus human providers.
In two of the four studies, we simply show the treatment groups an infographic, telling them that humans' decisions can be influenced by a variety of cognitive biases, so recency bias or confirmation bias, for instance. Then in the other two studies, we ask the treatment group to reflect on gender or age bias that they may have encountered in the past. And we ask the participants to choose between human or AI health care recommendations or whether they prefer care from an experienced nurse versus a less experienced nurse that is facilitated by an AI assistant.
Across all these studies, we consistently found that high bias salience reduces resistance to medical AI. And then in our last study, we want to examine the mechanism behind this effect, focusing on AI integrity. So it basically says, "When bias salience was high, how do participants feel about AI system?" And just as we predicted, when you trigger people's bias salience, they think AI has greater integrity. That is, the perceived fairness and perceived trustworthiness are higher.
So this essentially ties the story together, explaining the mechanism of this bias salience phenomenon that we observe. We show that we can, in fact, shift people's perceptions toward AI health care by making them aware of human biases.
Croft: In one of the studies you were just talking about, you had asked participants to write about the reason they chose either a more experienced consulting nurse or a less experienced nurse who was assisted by an AI assistant. And one of the comments you note in the study was from a participant who chose the more experienced nurse and said, "I would rather die from human error than a bug or glitch."
And that struck me as really getting to the heart of how strong AI aversion is for some people and the challenge in overcoming it.
Wang: Absolutely. … It shows that for some patients, they definitely have complete trust in human judgment, despite its potential fallibility. So generally speaking, from the study, we observe that there are participants like this one that prefer human providers. And they tend to-- in their reasoning, they tend to put a lot of emphasis on trust and empathy and human provider's clinical experience.
On the other hand, there are participants that prefer the nurse facilitated by an AI assistant. So it's human plus AI. And these participants tend to focus on the information and the knowledge and the functional aspects of health care delivery. And they also prefer having a second opinion or a different option.
So this is interesting, right? Not only we prime these participants with high versus low bias salience, but once they make the decision, we also ask them why. And then the reasoning-- just from reasoning, more of an exploratory type of analysis shows that patients actually have different emphasis when they search for health care-- when they acquire health care.
So this particular participant who would prefer to die from human errors over a bug or a glitch basically suggests that if you want to integrate AI into health care, then you must carefully balance the technology with clear communication. And hopefully, perhaps, AI in the future can be a complement to human care, but it's never a replacement of it. And I think that message needs to be clear.
Croft: That brings us to the next question I have, which is about what's at stake in finding ways to reduce the resistance many patients have to the idea of accepting the use of AI in their health care? I was struck again by a quote that was in your study citing a review article that was published in one of the leading peer-reviewed medical journals, Nature Medicine, that wrote: "AI is poised to broadly reshape medicine in the coming years."
So as AI becomes more prevalent, I guess we're getting to why does it really matter if people are accepting of AI or not? What's wrong if they just want human?
Wang: No, that's fair. I think especially there are a lot of challenges that the Nature Medicine article also highlights, which includes gathering unbiased and representative data and training the AI systems fairly, given that the data may not be representative. So there are definitely challenges. And I think people's algorithmic aversion toward AI medicine on some level could be justified.
This said, though, the potential benefits are also profound, such as using data for diagnostics and personalizing treatment options. It also streamlines the health care delivery process, perhaps making wait times shorter or allowing health care to be more equitable or more accessible to everyone.
For instance, virtual health care can make health care more accessible. And it can also potentially even help with early disease detection or even drug discovery. So instead of just doing annual checkups and everything seems OK, you can actually kind of predict what your next set of numbers might look like as someone ages. And then, hopefully, you can catch or predict. Even though the numbers are OK now, but maybe five years down the road, this patient might encounter X, Y, Z problems.
So all of this, in theory, could be done and I'm pretty sure in some circles are being done already. And hopefully, this will broaden out. But for this to broaden out, patients need to be willing to try it, to be willing to be open to AI medicine. So I think these are the stakes as we try to find ways to reduce AI resistance.