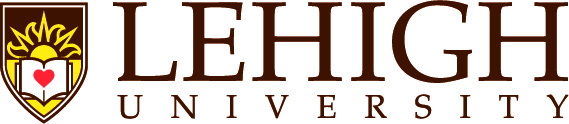
Image by iStock/DrAfter123
In this episode of Lehigh University’s College of Business ilLUminate podcast, we're talking with Burak Eskici about his perspectives on the role of generative artificial intelligence (AI) in business.
Eskici is a teaching assistant professor in the Decision and Technology Analytics (DATA) Department at Lehigh's Business School, where he co-directs the Computer Science and Business Program. His research interests include quantitative and computational methods; the development, application, and ethical use of artificial intelligence; systems design and optimization; and organizational behavior.
Eskici spoke with Jack Croft, host of the ilLUminate podcast. Listen to the podcast here and subscribe and download Lehigh Business on Apple Podcasts or wherever you get your podcasts.
Below is an edited excerpt from that conversation. Read the complete podcast transcript [PDF].
Jack Croft: One of the courses you teach at the business school is Foundations of AI for Business. From what you've seen, how is generative AI already changing the landscape in business? And let's start with, being optimistic, for the better.
Burak Eskici: So far, the AI, one thing is it is developing so rapidly. I designed this course on the Foundations of AI for Business throughout the summer. And when I designed some of the topics, for example, AI agents were not possible at that time. We were [in] discussions. Is it possible or not? Is it going to come in a year or two? But now we have AI agents within three months.
So the development is kind of exponential. This is why most of the benefits in terms of productivity are most on the personal level at the moment. We are using generative AI to maybe draft our emails, draft some documents. We use generative AI to personally write some code, debug or fix our code, do some data analysis. These are definitely helping at the individual level to save some time and come up with better quality product as well.
The issue at the business side is we haven't figured that out yet at the organizational level how to transfer or convert personal level gains into organizational or enterprise gains as well. So there's a big race, and this is kind of the big shift, I believe it's going to happen in the upcoming years. Some businesses, some companies are better in that sense, trying to develop these enterprise level gains.
At the moment, people are gaining some productivity efficiency gains at the individual level. This might result in the company level efficiency gains or more productivity, but we don't know how it's going to play out in that part as well.
The second part is we are still at the level of assistance-level AI. For example, we are asking AIs or the generative AI tools to assist us in some of the jobs that we are doing, some of the tasks we are tackling. But with the agentic systems, or the AI-based agents, coming into play, it will be a big kind of a paradigm shift. Then we will be able to say, "This is the data, this is the file, this is the topic, go and work on this report for me." And then … that AI agent is going to be able to search the web, download some stuff, look at the data analysis, and come back with the first draft. So this will be a big shift when we have these kind of agents, which is already some tools are available as of a couple of weeks ago. And we are going very fast in that direction as well.
Croft: Things are obviously moving, I think exponential was a good description of how rapidly it has been changing. So what are some of the downsides or potential downsides you've seen? And particularly, I guess in the area of ethical issues that are being raised.
Eskici: To begin with, for the ethical issues, all of these large language models or these generative AIs have been trained with existing data sources, the text, whatever we have on the internet, existing books, Wikipedia, anything you can think about this. And this has lots of legal disputes, who owns the rights and everything. I will just put that aside.
But whatever is the humankind, we have biases, some prejudices, or anything that in existing baked into the existing data is actually in the generative AI tools. So whatever we have as a humankind, we have biases, everything is reflected whatever we are getting from the generative AI tools as well. So it's a big deal. It's a big problem.
At the moment, we are using this as an assistance technology. We still are the decision makers and everything, but the moment it shifts, we don't have maybe more power or control to the AI assistance, then it will mean more bias at the level, whatever we have in the existing data, which is kind of a big problem.
The second one is these tools are a little bit black box. Explainability is a big issue in the AI at the moment. Now, because of the technical kind of details, the deep learning models, this large language models, it is really hard to interpret why it is giving the exact output. So we cannot go back and fix it very easily. This is why this black box part is quite challenging to use it in any decision-making process or other parts.
The third part is I think it's kind of dangerous enough that these large language models are working very well right now. Maybe in terms of quality, in terms of accuracy, especially, they are performing, let's say, at 90%. When they are at 95%, for example, it will be enough. It will be sufficient for us not to pay attention to quality of the output or the accuracy of the output, right?
So then when we stop paying attention, the accuracy and the quality, then we will end up lots of wrong results or difficult kind of problematic outputs that we will be using in corporate decision making, personal decision making, and so on and so forth, that this quality assurance of the large language models or the generative AI is going to be a bigger problem in the near future.
And unfortunately, we don't have a policy kind of framework at the moment. The technology is developing so fast. And there is a mismatch in terms of who is developing technology and who is supposed to provide the policy infrastructure. They don't speak the same language. This makes a big problem how we will have a good policy infrastructure that will enable responsible use of AI or responsible development of AI is a bit concerning, actually.
Croft: And I think that does get at a policy area, which is part of your background, but it's that question of how we as a society manage to encourage the benefits, the good things that you've talked about, and reduce the potential harms and the ethical problems that arise?
Eskici: In this case, for example, one thing I see in my students or in myself as well, I no more kind of draft something from scratch. This is concerning because when we think about something, when we try to write something on a blank page, it is the moment we struggle. It's a challenging task. But that kind of challenge also pushes us to think deeply, try to come up with better ideas.
This is the process of creating something new. The moment we delegate this to a generative AI or a computer program, slowly, slowly, maybe fast, I don't know, we will lose our skill to write something good or creative. Similarly, I kind of suspect in a couple of years, people stop writing emails or stop doing anything, which is going to be concerning at one hand. And I don't know how it will impact, how it will kind of play out in the near future.
Because I gave this example, it's similar to when calculators came out, right? The mathematicians or the mathematics teacher kind of protesting, … "You shouldn't use calculator." They were concerned about people are not going to be able to perform mathematics. It didn't turn out that way. It was kind of very useful for a certain extent, I believe. So it can also turn out in that way as well, but it is a little bit kind of it can go in both ways, I feel like.