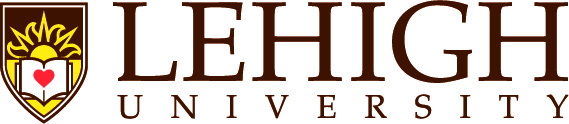
Image by iStock/gmast3r
In this episode of Lehigh University’s College of Business ilLUminate podcast, we are speaking with Kofi Arhin about his research regarding how AI may help make the hiring process more fair and equitable.
Arhin is an assistant professor in the College of Business Department of Decision and Technology Analytics (DATA). His research interests include artificial intelligence design and implementation, information security, ethical issues in information systems, human-computer interaction, and web technologies.
Arhin spoke with Jack Croft, host of the ilLUminate podcast. Listen to the podcast here and subscribe and download Lehigh Business on Apple Podcasts or wherever you get your podcasts.
Below is an edited excerpt from that conversation. Read the complete podcast transcript [PDF].
Jack Croft: I think it might be helpful to talk about one of the studies you've done recently - and you've done some other work in this area as well - looking at this question of how AI can be enabled to help make the hiring process more fair and equitable. You had talked about when you feed large amounts of data into AI for something like hiring practices, the human biases that went into those hiring practices over the years gets transferred in there with it.
So if you could talk a little bit about that history of what the experience has been in human resources (HR) with the use of AI, some of the problems that have been highlighted quickly, and then what it is that you're looking at that might be able to make things better.
Kofi Arhin: The challenge, from literature and from reports, has been that these AI tools are learning from data and these data contain historic human decisions that may be discriminatory. For example, research has shown that the way people look, whether it's the color of your hair, your skin color, even the way you dress sometimes, can impact a hiring manager's decision to employ you or to move you to the next stage of the personnel selection process.
Other studies have also looked at how language impacts the interview applicants, or job applicants. And there are several standardized tests that have also been shown to affect underrepresented group members negatively. And so in the U.S., the Civil Rights Act of 1964 and the Uniform Guidelines [on Employee Selection Procedures] provide guidelines on how people who apply for jobs should not be discriminated [against] based on age, race, religion, gender, and so on. So going back to hiring with AI, when you train AI models on historic human decisions, you might be replicating or automating the biases in those decisions. The solution to this challenge, especially in hiring, has been identifying the human biases and trying to address them.
Let's assume that a company has identified that their AI system could be discriminatory. What they have then to do is to go back to the data and try to take out information that may be highly correlated with a particular subgroup, like race or gender or religion or something, so all the features or attributes in your data that are correlated with these different protected classes.
The challenge, I argue together with my co-authors, of course, is that when you start manipulating the data, you have to do it for-- so let's talk about race, for example. If you're doing it for Black applicants, you have to do it for Hispanic applicants, you have to do it for Indigenous applicants, and so on. There are so many groups in race alone. Then you go to gender. You have to make sure that people with different gender identifications are being treated fairly. And then you go to religion, and so on and so forth.
In the end, you're going to have a data set where you have removed a lot of information about underrepresented group members or you have tried to manipulate the data in such a synthetic way that it is no longer representative of society.
When you train an AI model on this data, yes, it might give you, in the short term, fair outcomes. But in the long term, you're going to have an AI system that has a lot of information about majority group members, because you are not taking their information out of the system, and it knows very little about underrepresented group members. That's one setback.
Two, in manipulating your data or training your AI to be more fair, there's a potential that you might be discriminating against the majority group members. … Let's look at gender in terms of majority and underrepresented. So let's say we have a job vacancy where male candidates dominate the application pool. They've dominated the successful hiring process over a long period of time. I'm assuming two gender types. And so the female-- if they are the underrepresented group, now you have to treat them differently if you want your AI system to address that historical human bias.
… What you have to do then is to adjust the system to treat female applicants separately from male applicants. In doing that, you can run into a potential legal challenge where you are discriminating against the male applicants. Remember, the law applies to all. The law is not only meant to treat a particular group fairly and another group unfairly, right? And so there could be legal challenges.
What I propose in my study … is I argue, hey, let's keep all the representation. Don't even touch the data because it represents something about these applicants that are important. So keep all the information in the data. Do not remove words. Do not remove attributes or features that you find might be highly correlated with subgroup membership. Let's find other sources of information.
Let's assume that we are training the AI on our data. We are training the AI to model historic hiring manager decisions. In my study, we argue, let's train the AI on other sources of information that can help us identify the best candidates in our pool. So don't just rely on historic hiring manager decisions in the past. Train the AI to learn who a good applicant is from other external sources.
When you've done that, bring it together and then compare what the AI has learned from outside the organization to what your historical decisions have been. Find the intersection between the outside knowledge and the internal organizational data, and then pick the applicants at the intersection of these two sources.
The rationale behind that is training your AI on external sources introduces some objectivity. Well, that's our hope in the study, that it introduced some objectivity in the way the AI makes decisions. This is not to say that AI systems are not objective, but whatever decision the AI is making is relative to the data that belongs to the organization, right? So don't just rely on the organization information. Take information from external resources. Find the intersection between that, and then your AI system is equipped with enough knowledge to know who a good applicant is.
So we are still using the historic hiring manager decisions, but we are also using external knowledge, and we are finding the intersection between the two. And we find that when we do that, our AI systems are fairer.