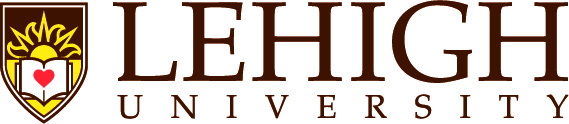
In this episode of Lehigh University’s College of Business ilLUminate podcast, we are speaking with Sterling Yan about whether machine learning methods can actually boost investment performance over results obtained through traditional models. Yan holds the Joseph R. Perella and Amy M. Perella Chair in Finance in Lehigh's College of Business. His main research interests include asset pricing, institutional investors, mutual funds, hedge funds, short selling, and liquidity.
He spoke with Jack Croft, host of the ilLUminate podcast. Listen to the podcast here and subscribe and download Lehigh Business on Apple Podcasts or wherever you get your podcasts.
Below is an edited excerpt from that conversation. Read the complete podcast transcript.
Jack Croft: How prevalent has machine learning become in predicting investment performance?
Sterling Yan: I think it is very difficult to get a precise idea on this, but if you are a large asset manager, if you are an asset manager with a decent size, I don't think you can afford not to look at machine learning nowadays. Having said that, most of them have explored machine learning in their investment process. The extent to which they actually use machine learning in their investment process is an open question.
Croft: So is it more of a case … where you have investment and fund managers use it as a supplement to what they do, as opposed to relying on it to make decisions?
Yang: That's a very good way to characterize the role of machine learning in the investment process nowadays. So [there] are two categories. Some of the fund managers, perhaps, develop a fund, entirely do it on the idea that the maximum process is going to be done with machine learning strategies. But most of the funds, traditionally, are using machine learning strategies as a supplement to help with their existing investment strategies and methodologies.
Croft: There have been a series of studies over recent years, asset pricing studies, that usually find that machine learning can double or even triple stock market returns delivered by traditional models. What was it that led you and your three academic colleagues from other universities to examine whether that was actually the case?
Yan: There are two broad reasons. One is sort of a general reason why we are a little skeptical about this finding and the other is a specific reason. So let me talk about the general reason first. There's actually a theory in finance and that is, the market is pretty efficient. Now the market is not always efficient, but the market is pretty efficient. That's one default theory of the financial market. When the market is efficient, by the way, stock returns are not very predictable except that one is predictable because they are risky.
And the idea why the market may be quite efficient is built on the idea that if the market is not efficient, then smart investors will try to exploit that and the simple process of exploiting market predictability is going to make the market more efficient. That's why there is the default theory saying that the market should be pretty efficient. That's one general broad conceptual reason why one can be skeptical about a very large magnitude of predictability documented by previous studies.
A specific reason has to do with the design of the studies, in these recent studies, a particular design choice. And it turns out what happened was that most of the recent studies use anomaly variables, investment signals, that is that word discovered in the more recent time period, and they assume that real-time investors in the 1960s, many decades ago, were able to be aware of those predictors and use them to be able to predict returns. So there's a hindsight bias, in some sense, in that choice of methodology. I can elaborate on that if you want to, but there is a hindsight bias in some sense that is--
Croft: Yeah. I do think that's interesting. And I think it would be interesting for our listeners to understand that a little better as well. This idea that in looking at these anomaly variables that have been developed, say, from the '90s onward and then applying them back to the '50s or '60s or '70s, that there's an underlying assumption that investors at that time in the '50s and '60s and '70s were aware of these variables that weren't actually identified until decades later.
Yan: Exactly. … This has to do with the field of finance being different from the other fields … where machine learning and artificial intelligence have been very, very successful. For example, artificial intelligence can play chess much, much better than human beings are able to. And there are many, many other areas, image recognition, cars driving themselves. There are many, many other areas where artificial intelligence is extremely successful.
But there are some fundamental differences between finance, between return prediction, trying to predict future returns from other applications like I just mentioned. Meaning that in finance, in order for machine learning, artificial intelligence to be successful, one of the things that's necessary is that you ought to have an abundant amount of data, a large amount of data for the machine learning, for the artificial intelligence to be able to learn from. That's actually not the case in finance. We can't artificially generate new data—come up with images or let a car drive itself or let the artificial intelligence play chess games to create millions and millions of new chess games.
In finance, we can't generate data that way. The market goes down today by 1% or it goes up at 1%. That's the data we have. We can’t artificially generate a new set of data where today, the stock performed differently. So the amount of data is relatively limited in the finance area. And there are some other differences where finance is different, and that is one of the reasons why machine learning strategies may not be as successful as one would expect.
Croft: Now as simply as you can, if you could talk about the methodology you used in your study. And then I think what most people are most interested in, of course, is kind of the bottom line. What were the main findings of your study?
Yan: What we ended up doing was, let's take a real investor's perspective. Let's assume we're in the 1950s and '60s. We didn't know what was going to happen in the 1980s, right? We didn't have the hindsight. And therefore, we're going to look at the data we have. We're going to construct a bunch of strategies. We're going to call that a universe, OK? We didn't know in the '50s, '60s which one was going to work exposed. So what we're going to do is we were going to learn from the universe.
So what we end up doing is we tried to simulate that process. We construct a universe of signals, over 18,000 of them. And assuming the investors were able to use machine learning strategies in the '50s and '60s and try to learn something from that universe, and then use that to enhance our implemented investors strategy. And then we evaluate the performance of that strategy.
So what our main findings are that yes, we find that machine learning strategies do work in the sense that they can enhance your investment performance, but not to the extent that was documented in the recent studies that we just talked about. So the magnitude of the investment performance improvement is substantially smaller than what has been documented and again, for reasons of the limitations of their methodology.
Croft: And in terms of kind of looking at a crystal ball into the future, as machine learning continues to grow more sophisticated, how do you think the role of the human fund managers will change in the future?
Yan: That's a very good question because we can never underestimate how fast or how much the technology is able to change, right? So machine learning strategies themselves will become more sophisticated, perhaps will be reaching … a stage where it can do things that are not thought possible … today, even in the area of finance. So that's possible. But those fundamental characteristics of financial markets that I have talked about maybe two or three times already in this podcast, those things will not change very quickly.
And as a result, the potential of machine learning strategies in the area of return prediction, in my opinion, will continue to not be as impressive as what we would expect or we would have hoped. And because of that, I think human investors, human managers will continue to play an important role in the investment process.
And in the future, maybe some sort of a combination between human beings and artificial intelligence. That may be the optimal model going forward. The machine learning will likely ... play an increasingly important role, but there will not be any substitute for human involvement in this process.